Since 1968, the World Health Organization (WHO) Programme for International Drug Monitoring (PIDM) has existed to globally focus the analysis, reporting, and monitoring of adverse drug events. The main goal of this international pharmacovigilance program is to increase patient safety with regard to the use of medicinal products by providing reliable information to the 151 member countries.
Despite this centralized pharmacovigilance system, adverse drug events (ADEs) continue to occur daily and are considered a severe public health issue. According to the U.S. Centers for Disease Control, in the United States alone, ADEs cause approximately 1.3 million emergency department visits and 350,000 hospitalizations every year. The constant introduction of new pharmacological agents and the increased number of ADEs render it crucial for pharmacovigilance teams to be reactive.
The primary mission of a pharmacovigilance process is “the detection, assessment, understanding, and prevention of adverse drug effects or any other possible drug-related problems”. Today's pharmacovigilance processes are unoptimized due to heavily manual workflows. Pharmacovigilance teams are currently managing adverse drug events reports manually which creates efficiency and scalability challenges.
“Pharmacovigilance processes must evolve and implement modern data science and medical informatics methods to meet the ever-growing needs for efficiency, transparency, and reactivity.” Dr. Louis Letinier Medical Director & Co-Founder Synapse Medicine
The Main Pharmacovigilance Challenges
Most ADEs are identified during clinical trials before a drug is introduced to the market. However, despite all the safety measures taken during these studies, not all ADEs can be identified. This is due to the fact that clinical studies are often conducted using a limited number of subjects, which cannot represent the entire population in terms of age, health background, etc. Moreover, clinical trials do not allow the detection of long-term adverse effects.
The three main pharmacovigilance challenges are:
- Addressing the steady increase in adverse event reports, particularly from patients.
- Improving the responsiveness of pharmacovigilance systems during health crises.
- Integrating new technologies adapted to the world of big data and unstructured data management.
Superpowering Pharmacovigilance with AI
Dr. Louis Letinier Medical, Director and Co-Founder, together with Julien Jouganous, Lead Data Scientist at Synapse Medicine, authored a scientific publication entitled «Artificial Intelligence for Unstructured Healthcare Data: Application to Coding of Patient Reporting of Adverse Drug Reactions» published by the American Society for Clinical Pharmacology & Therapeutics (CPT).
In this publication, they explore ways to facilitate the monitoring of adverse drug event reports using artificial intelligence. The article was featured in the August 2021 issue of CPT. This section summarizes the research publication’s methodology and findings.
“The future of pharmacovigilance will always be supervised and interpreted by experts, but the time-consuming routine work will have to be facilitated by the machine.” Dr. Louis Letinier Medical Director & Co-Founder - Synapse Medicine
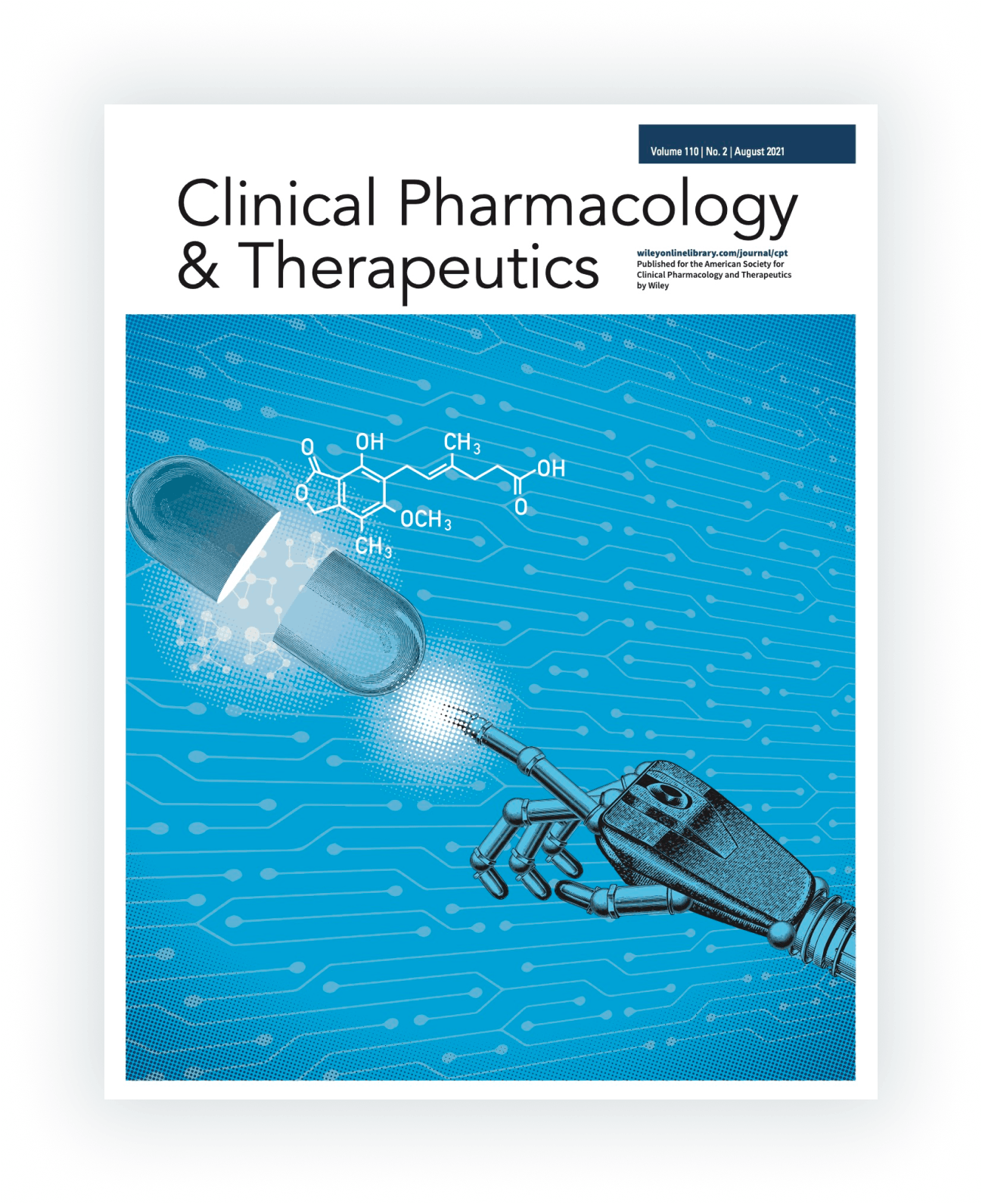
Benchmarking Machine Learning Models
In order to effectively benchmark the selected machine learning models, it was essential to process a significant data set. For the task, the data used came directly from reports from the Bordeaux Pharmacovigilance Centre via the French national ADE reporting website. It gathered 2058 reports from March 1st, 2017 through February 28th, 2019.
The coding of ADE used the MedDRA standardized medical terminology developed by the International Council for Harmonization of Technical Requirements for Pharmaceuticals for Human Use.
The study evaluated the main types of machine learning models that are commonly used in text classification: conventional machine learning models, FastText, Deep learning models, regular expressions, and hybrid models.
The machine learning models were trained on 90% of the data set (training set) and the computing evaluation metrics were tested on the remaining 10% (test set).
The best-performing model was selected based on two performance metrics used to evaluate classification models: the area under the curve (AUC), and F-measure.
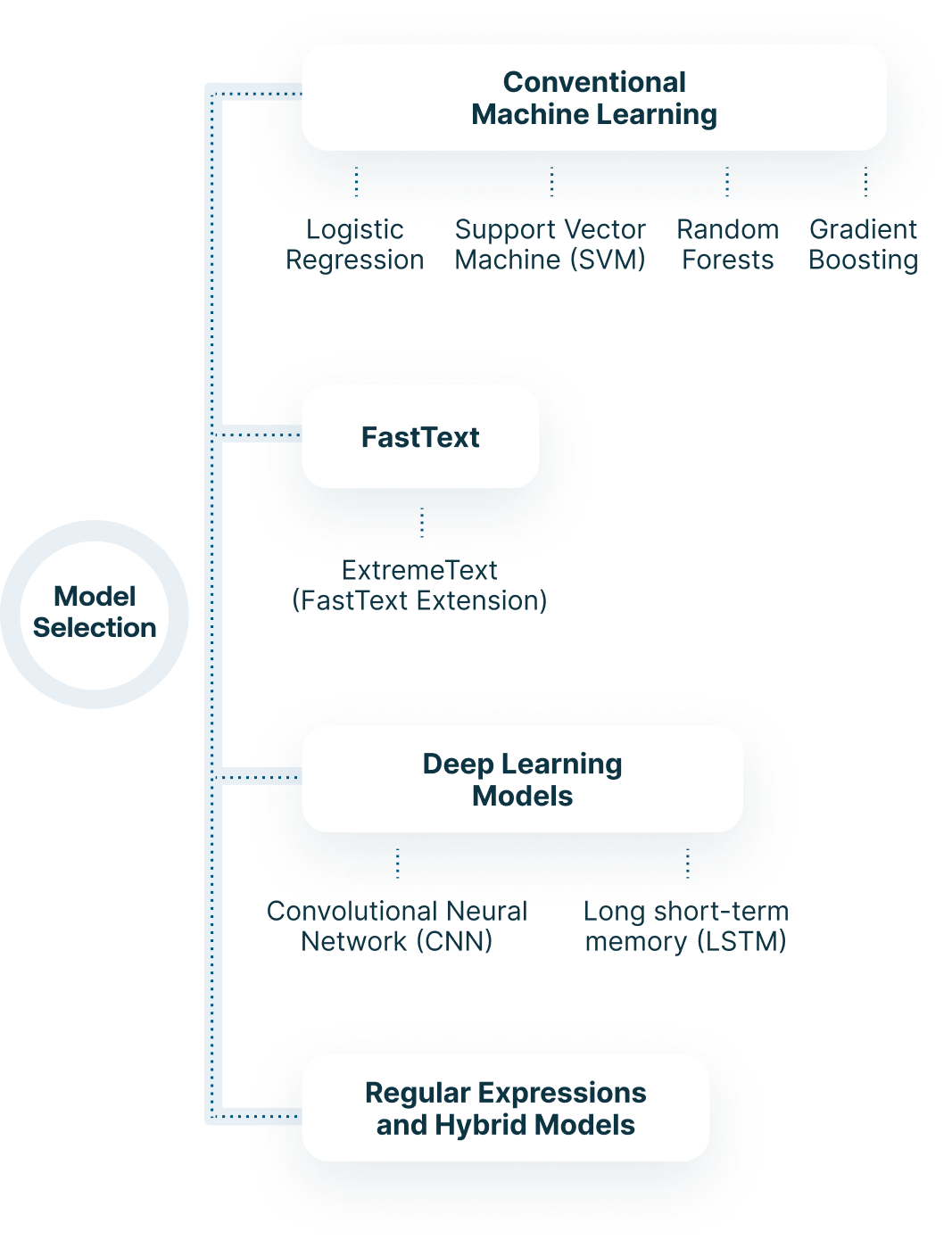
Building the Optimal AI Workflow
The objective of the «Artificial Intelligence for Unstructured Healthcare Data: Application to Coding of Patient Reporting of Adverse Drug Reactions» scientific publication was to develop an automated system that allows the coding of ADEs and their associated seriousness from free text sources. It was therefore critical to implement a process to identify and validate the best AI pipeline. Illustrated below is the pipeline that is currently being used.
1. An adverse drug report is filed using an online form with a free text field.
2. A PDF is generated to consolidate the patient case report.
3. The data from the PDF are extracted and structured.
4. The machine learning models perform a pre-annotation of the MedDRA terminology and the severity assessment.
5. Pharmacovigilance teams then either validate or edit the case report.
6. The report is transferred to the BNPV. (French National Pharmacovigilance Database.)
7. The edited case report is injected back to improve the machine learning models.
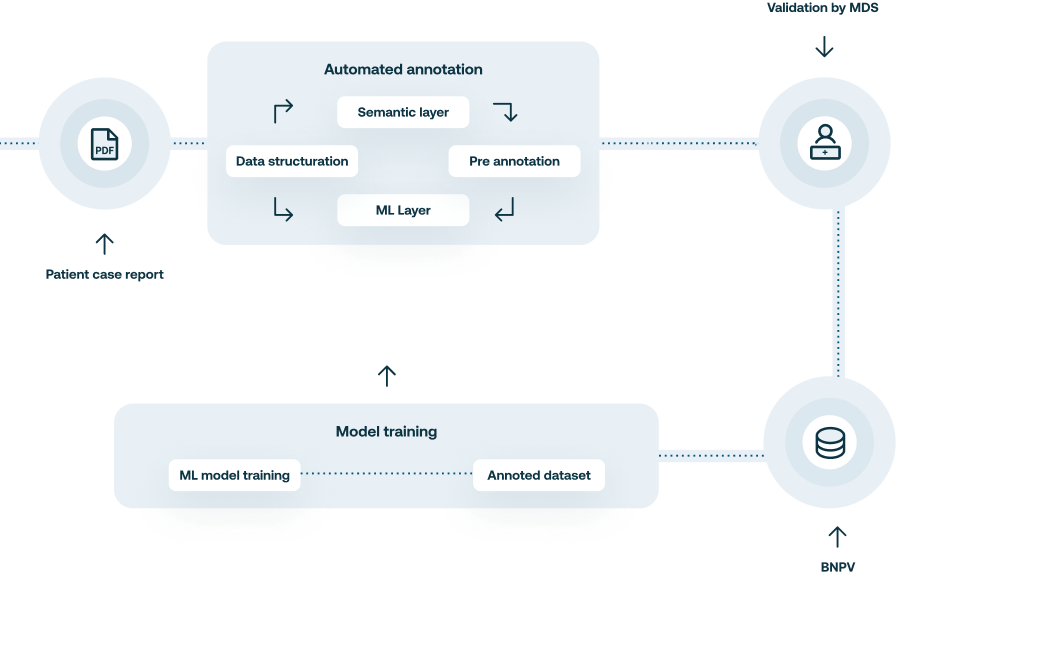
Enhancing the Medication Shield's Performance
A second research publication was authored by Synapse Medicine's medical and data science teams to better understand the performance of the Medication Shield's AI models. It was published in Drug Safety from The Official Journal of the International Society of Pharmacovigilance [ISoP] on May 2022 and titled "Validation of Artificial Intelligence to Support the Automatic Coding of Patient Adverse Drug Reaction Reports, Using Nationwide Pharmacovigilance Data".
The publication is a validation study of the medication shield on a French national scale. Data from 27 CRPVs were used (10,500 ADE reports in total) for learning and internal validation of the models.
Furthermore, external validation was performed on a 28th CRPV (1,200 ADE reports in total). The results of the best model retained (LGBM) showed an AUC of 0.97 and an F-measure of 0.80.
It's important to note that these results were consistent in the external validation.
Thus, this second research publication clearly showed that the performance of the Medication Shield improves with an increase in the volume of data and that the model is now sufficiently robust to be transposed to external data.
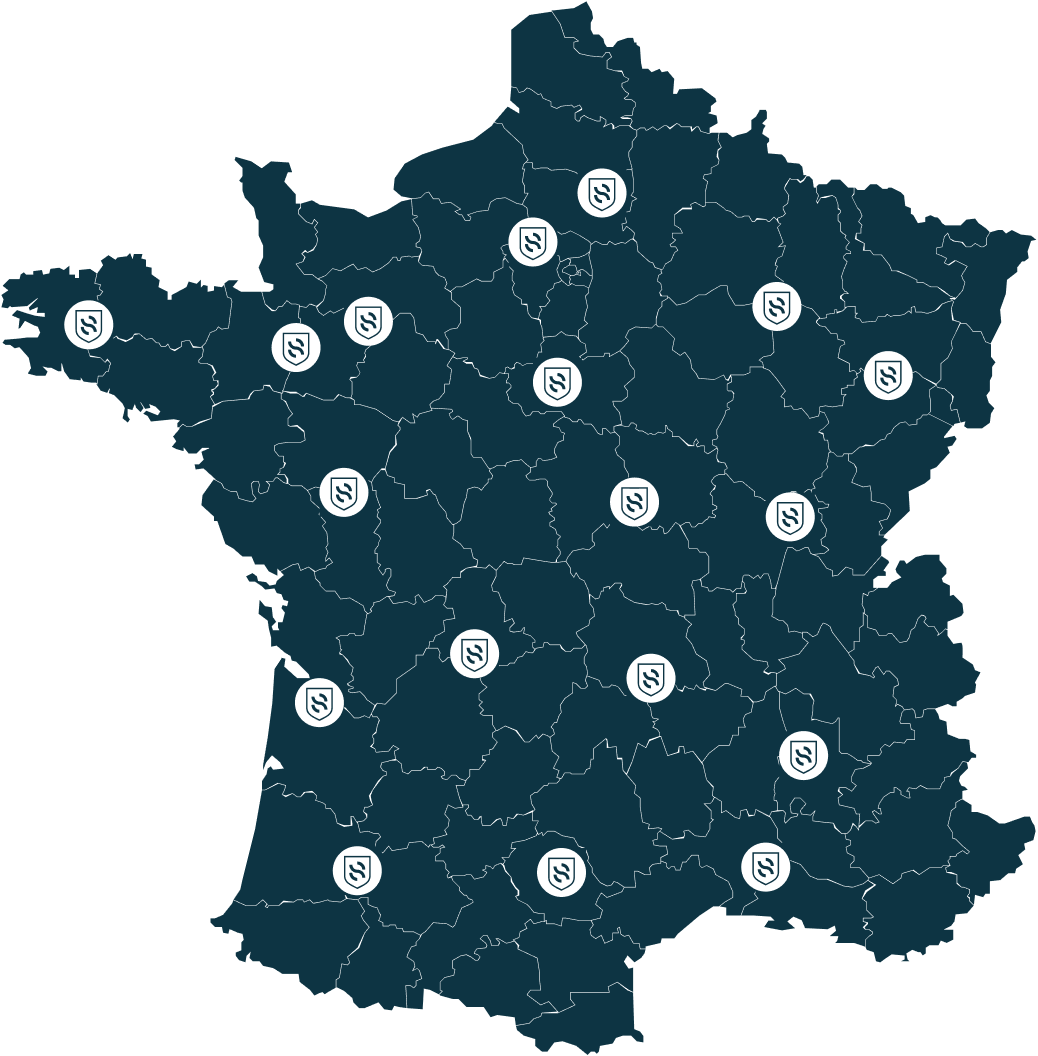
Automating ADE Report Monitoring
Nowadays, the reporting of an ADE is usually done online by healthcare professionals or patients by simply filling out a form. Processing this free text is a challenging machine-learning problem as it includes a combination of structured and unstructured data.
Afterward, the collected reports are managed manually by pharmacovigilance teams which then have to prioritize themselves.
Without an AI acting behind the scenes to filter the ADE reports' severity, the pharmacovigilance system is inefficient because of its limited ability to detect new safety signals easily and in real time.
“By applying automation to PV, we are clearly demonstrating the ability to improve patient safety and reduce time-consuming manual tasks for pharmacovigilance teams.” Dr Louis Létinier - Medical Director & Co-Founder Synapse Medicine
The impact of an automated ADE reporting system:
- ADEs are collected and filtered by severity with an automated system.
- Real-time detection of new pharmacovigilance signals.
- Enhanced population health safety.
- Time-savings for healthcare professionals which allows more time for other pharmacovigilance activities.
- Standardized ADE coding taxonomy across the pharmacovigilance system*
*In pharmacovigilance, ADEs are coded based on the MedDRA terminology, which has its limits. One of the main drawbacks is the management of synonyms in medical terminology. e.g., tiredness and asthenia. An automated ADR coding system helps to standardize these terms and facilitate signal detection.
Automating Pharmacovigilance for the COVID-19 Vaccination Campaign
During the initial COVID-19 vaccination roll-out and continuing through today, it has been necessary to remain on high alert for adverse events potentially related to the COVID-19 vaccines. Using the learning from the scientific publication « Artificial Intelligence for Unstructured Healthcare Data: Application to Coding of Patient Reporting of Adverse Drug Reactions'', Synapse Medicine's teams developed an innovative solution to safeguard the vaccination campaign in France.
The technology is named "Medication Shield" because it acts as a shield to protect the population.
In 2017, after various health scandals related to medications, including Mediator and Levothyrox, the French health authorities deployed an online portal dedicated to Adverse Drugs Event (ADEs) reports. This platform facilitates the reporting of ADEs, their identification signals, and the distribution of information to nationwide pharmacovigilance teams. Soon thereafter, in 2019, Synapse Medicine, the ANSM (French National Agency for the Safety of Medicines and Health Products), and the Regional Pharmacovigilance Centers began collaboration on a research project studying the application of artificial intelligence to pharmacovigilance case management.
A French governmental institution, the ANSM is responsible for assessing health risks related to healthcare products. As such, the ANSM is closely involved as soon as a new drug reaches the clinical trial stage. The French Regional Pharmacovigilance Centers collect ADE reports and assist in diagnosing and managing any pathology related to drugs. Together, they constitute a pharmacovigilance network that supports prescribers, alerts health authorities, improves the quality of drug management, and protects patients.
The ANSM sought out Synapse Medicine’s Medication Shield technology to facilitate the management of ADE reports by national pharmacovigilance teams.
The Medication Shield was deployed nationwide for one purpose: to facilitate the mission of pharmacovigilance teams and help them be more responsive in managing ADE reports, especially when severe and unexpected.
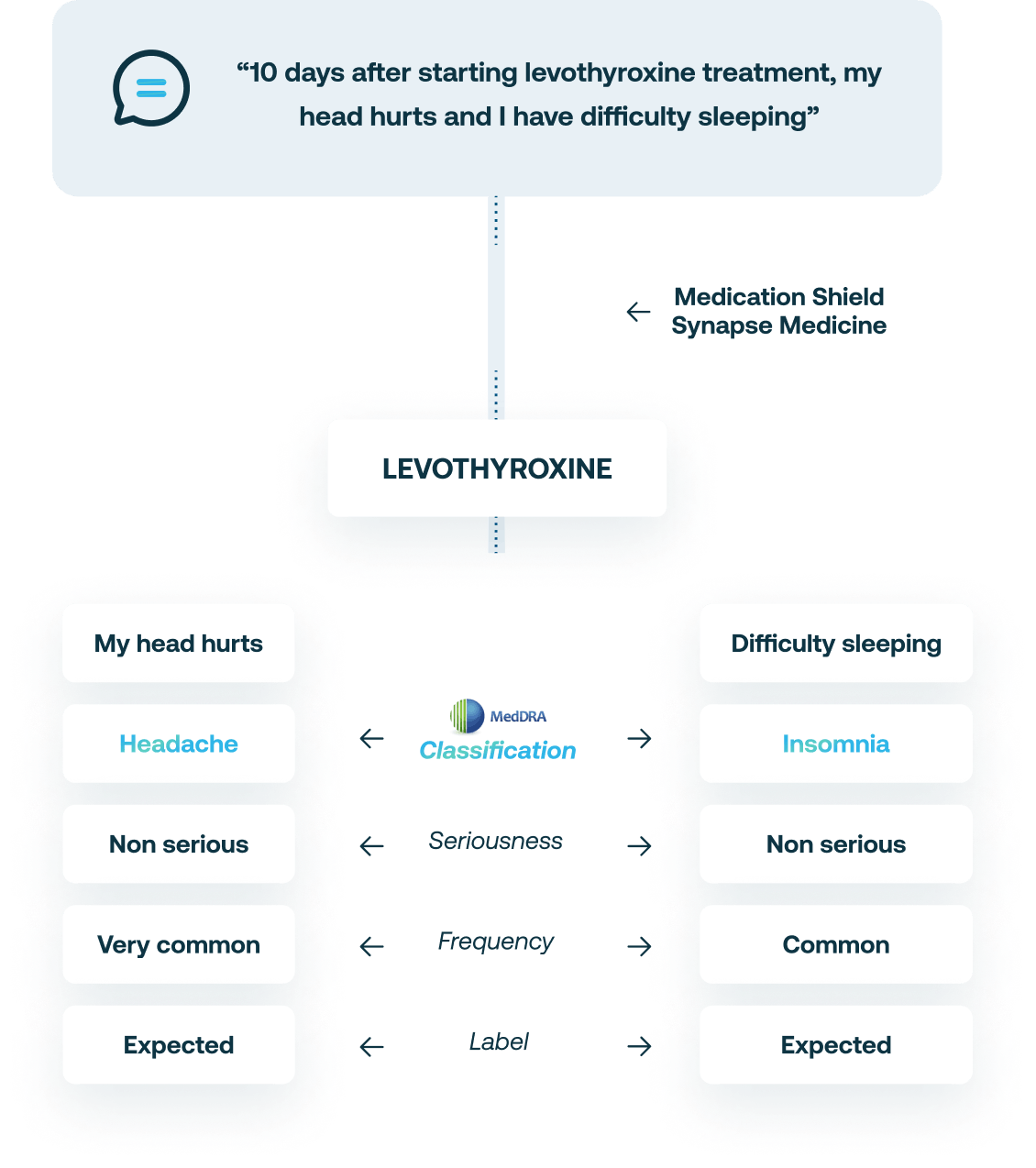
The Medication Shield Performances
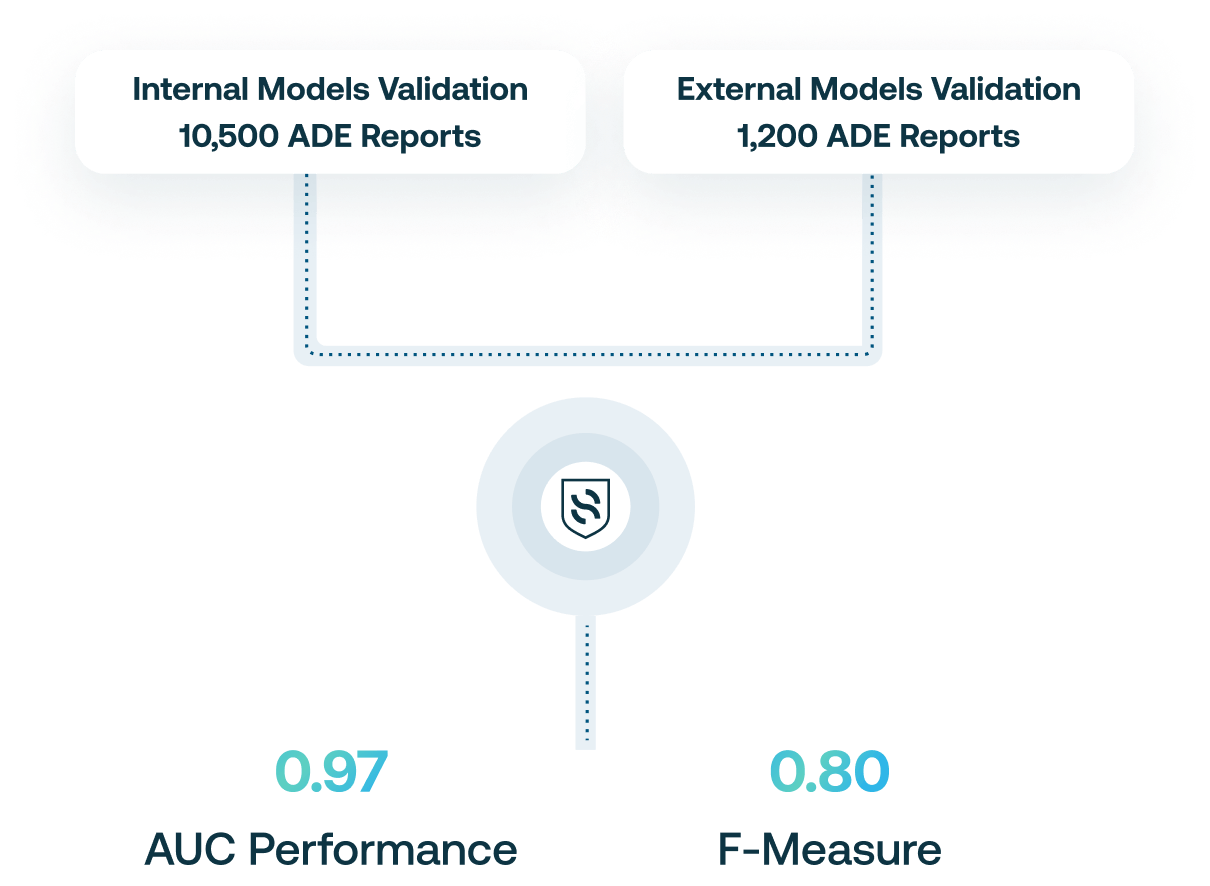
Enhancing the French Pharmacovigilance System
1. Reporting potential ADEs from COVID-19 vaccines
The reporting portal implemented by the French Ministry of Health allows the Regional Pharmacovigilance Centers (CRPVs) to be notified in real-time of COVID-19 vaccine-related ADEs detected by healthcare professionals, patients, or the pharmaceutical industry.
2. Automating the Pharmacovigilance with the Medication Shield
Synapse Medicine’s Medication Shield is used by CRPVs and the ANSM to manage COVID-19 vaccine-related ADE reports.
3. Facilitating Pharmacovigilance Team Jobs
The CRPVs are able to prioritize pharmacovigilance signals and therefore focus on the most severe ADE cases.
4. Improving Health Authorities Response Time
Using the Medication Shield, the ANSM, in collaboration with the EMA (European Medicines Agency), can decide if corrective measures must be taken such as notifying the public by adding an ADE to the medication leaflet or whether or not to withdraw a drug from the market.
Strengthening Pharmacovigilance with the Medication Shield
Synapse Medicine’s artificial intelligence solution for pharmacovigilance facilitates the management of adverse event reports.
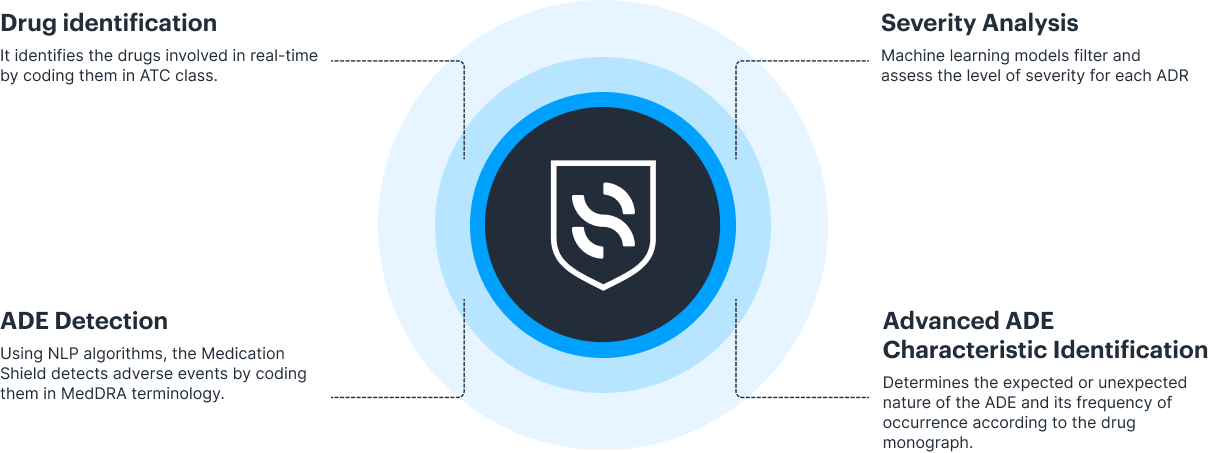
An Award-Winning Technology
The ANSM sought out Synapse Medicine’s Medication Shield technology to facilitate the management of ADE reports by national pharmacovigilance teams.
The Medication Shield was deployed nationwide for one purpose: to facilitate the mission of pharmacovigilance teams and help them be more responsive in managing ADE reports, especially when severe and unexpected. Synapse Medicine’s Medication Shield technology was the winner of the Prix Galien France 2021 in the digital health category. It was also nominated for the Prix Galien International 2022.
Conclusion: Automated Pharmacovigilance Enhances Public Health Safety
As discussed throughout this article, ADE reports are increasing exponentially each year, and few pharmacovigilance automation technologies are being developed around the world to prevent this increase. Using algorithms streamlines the process by discovering hidden patterns in data sets and facilitating the surveillance of pharmacovigilance signals.
The automation of pharmacovigilance offers a promising solution to this population health safety issue.
The main pharmacovigilance automation benefits:
- Improves population health safety
- Real-time detection of pharmacovigilance signals
- Precise visibility of the benefit-risk balance
- Standardization of ADE coding (MedDRA)
- Deployable worldwide
- Time-savings for pharmacovigilance teams